
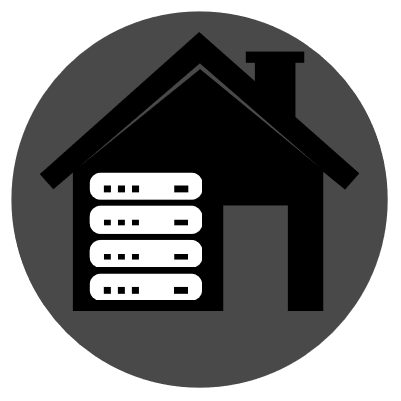
Yup; hopefully there are some advances in the training space, but I’d guess that having large quantities of VRAM is always going to be necessary in some capacity for training specifically.
also at beehaw
Yup; hopefully there are some advances in the training space, but I’d guess that having large quantities of VRAM is always going to be necessary in some capacity for training specifically.
Yup; hopefully there are some advances in the training space, but I’d guess that having large quantities of VRAM is always going to be necessary in some capacity for training specifically.
So I’m no expert at running local LLMs, but I did download one (the 7B vicuña model recommended by the LocalLLM subreddit wiki) and try my hand at training a LoRA on some structured data I have.
Based on my experience, the VRAM available to you is going to be way more of a bottleneck than PCIe speeds.
I could barely hold a 7B model in 10 GB of VRAM on my 3080, so 8 GB might be impossible or very tight. IMO to get good results with local models you really have large quantities of VRAM and be using 13B or above models.
Additionally, when you’re training a LoRA the model + training data gets loaded into VRAM. My training dataset wasn’t very large, and even so, I kept running into VRAM constraints with training.
In the end I concluded that in the current state, running a local LLM is an interesting exercise but only great on enthusiast level hardware with loads of VRAM (4090s etc).
Interesting! Sakurai would say keep your params out of the code, so that you can easily tweak all params in one spot when balancing things. But maybe having all params in code is reasonable to handle when you’re a solo dev.
I don’t know anything about GPU design but expandable VRAM is a really interesting idea. Feels too consumer friendly for Nvidia and maybe even AMD though.